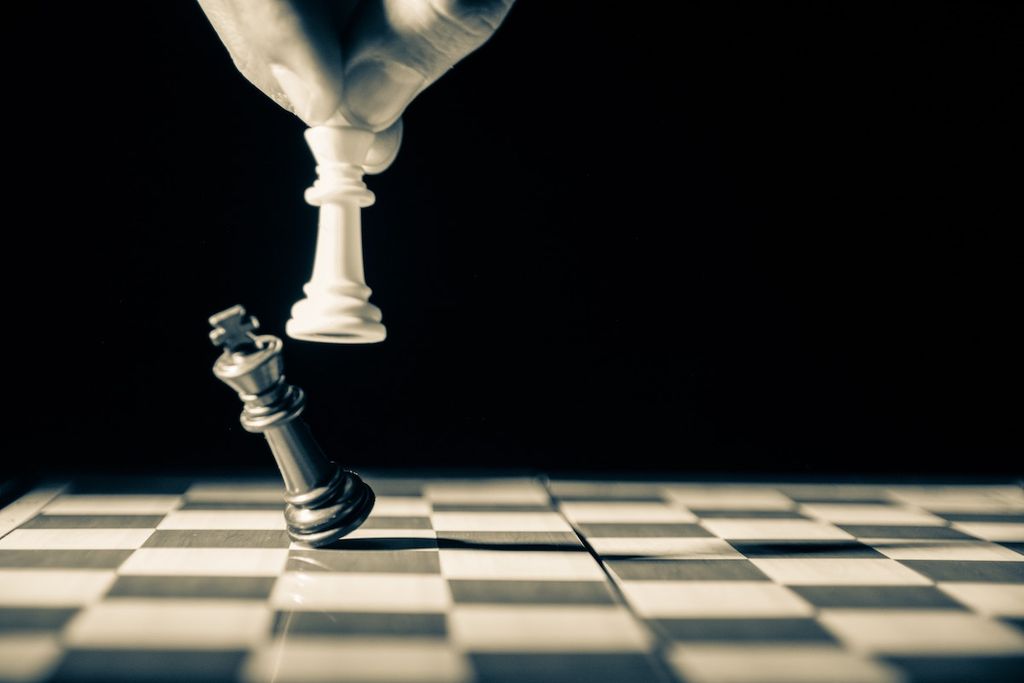
Can AI Have Its Cake and Eat It? Reducing Bias in AI Models May Not Always Be Desirable
This is the first blog in the AI Research and COVID: Journeys to Gender Equality and Inclusion series. The pieces from this series emerged from the “writeshop” organized by Gender at Work as part of the Data Science and Artificial Intelligence Research Program to Combat COVID-19, also known as AI4COVID, financed by the International Development Research Centre’s (IDRC) and the Swedish International Development Agency (SIDA). This was part of the final Gender Action Learning workshop held in Nairobi, Kenya, in February 2023.
In this first blog, Amelia Taylor, a Senior Lecturer in AI at the Malawi University of Business and Applied Sciences and researcher with the INSPIRE PEACH project under AI4COVID, raises the ethical dilemmas of trying to create unbiased and representative algorithms of women and men impacted by epidemics.
What I learned about gender biases from chess and communism
I grew up in Romania in the 1980s, under communism. Men and women were by definition ‘equal agents of production.’
During that time, I learnt chess from my dad from a young age. He taught me rules, strategic openings and endings. Despite never taking up chess professionally, I participated in some competitions in Romania and trained with a top-rated European junior, a girl who lived in a block of flats a few streets away from me.
Chess is an intellectual activity. Men and women can engage with each other on an equal basis: the rules are the same for both. But the top chess players in the world are men.
Garry Kasparov, the Soviet-born chess master, once said, “Some people don’t like to hear this, but chess does not fit women properly. It’s a fight, you know? A big fight. It’s not for women.”
Despite this view, women did excel at chess. Take Vera Menchick, the pioneering first and the longest-reigning Women’s World Chess Champion, and Judit Polgar, the Hungarian chess grandmaster who many consider the best female chess player of all time.
Now suppose you design an AI program that teaches and encourages women to play chess. Some studies found that women chess players generally are more risk-averse than men but that top women chess players play more aggressively. Other studies show that women tend to play differently when they know that the other opponent is male. The data to train the AI program needs to contain a large database of games that have been evaluated for their strength of strategy. Naturally, many of these games and positions would come from representative games played by male players.
But does that create a bias? You could answer yes, because the data is biased by design, and not many games played by women are part of the training set. Or, you could answer no, because women want to learn how to win against both men and women.
Regardless of your opinion, reducing the gender bias in chess data would not lead to an improvement in the ‘performance’ of the AI model aiming to prepare women to be chess champions, but can be important to creating a better atmosphere so young girls will be less intimidated and stay motivated to learn.
Now let’s look at a higher-stakes example. Suppose you want to train an AI algorithm to perform robotic surgery. Would it matter if the data to develop it comes mainly from male surgeons? Would that bias be undesirable?
The answer is complex.
I teach algorithms analysis and design. Algorithms consist of finite sequences of computable steps. These steps apply to an input that must satisfy some known conditions, called “pre-conditions”. The output of an algorithm also must satisfy a set of conditions, called “post-conditions” used to judge the quality of a solution.
No one hides these conditions or tries to remove them. They must be known and indeed are needed to write the internal steps of algorithms. Their presence helps reduce “noise” (any data that is either corrupt or cannot be interpreted correctly by machines.) But could these conditions help us in detecting biases too?
Gender bias is indeed an important aspect of AI. The above scenarios illustrate the need for clarity in defining the type of gender biases that, if reduced, might lead to more accurate AI models that produce fewer errors in their decisions or outputs from the data.
AI and gender in our GAL* project
As part of Global South AI4COVID program, the INSPIRE PEACH started in 2021 and brought together several institutions to analyse, compare and harmonise Covid-19 data in Malawi and Kenya. My role in this project was to understand and analyse Covid-19 data collected in Malawi along several dimensions including on the lines of gender and intersectionality.
We wanted to know specifically if more men or women become infected and/or die of Covid, and if surveillance data at points of entry to Malawi —both land and air —have a male bias in it. One study showed that males, of older age and from urban residence, were associated with increased Covid-19 morbidity and mortality. Knowing this bias could be positive and improve treatment and care for such groups.
During the height of the pandemic, measures such as partial lockdowns and closures of schools and markets were meant to mitigate the impact of Covid-19 on the population and reduce its spread. As a data scientist, I was looking for pre-conditions. I also wanted to know what kind of post-conditions could be expected.
One pre-condition was that men usually participate in large gatherings such as political rallies, whereas women did not. Another was that women especially (and also men) needed to spend more time to look after their children who were now at home, and as a result, women saw their incomes reduced. But, we later saw that the lockdowns resulted in damaging consequences — or post-conditions — for many girls and women. More young girls became pregnant during school closures and many never returned to school after the restrictions were lifted. In Malawi, women experienced increased sexual harassment. Also, Covid restrictions aggravated vulnerabilities and inequalities for women’s access to employment and economic power, which existed prior to pandemic.
It can be argued that decisions regarding preventive measures may solve some biases-–because men are at a higher risk for Covid, the decision to reduce public interactions makes sense. But these same measures may exacerbate other biases—for example, closure of school leads to more girls dropping out of school and other unexpected results. Lockdowns may have been effective in preventing contagion among men, but they had a large negative impact on women.
Reducing AI biases and increasing representativeness is not always desirable
Giving equal consideration to all possibilities is too demanding: machine storage and computing power are both finite and limited. Biases help humans make decisions easier by providing a starting point, an initial prediction regarding which choice to make. Similarly, AI algorithms usually use “heuristics” to achieve intelligence. Heuristics are rules of thumb, enabling someone to discover or learn something for themselves. In AI, heuristics are special rules that allow an algorithm to arrive at a solution quickly –- this may not be the best solution but a satisfying solution.
During COVID-19 in Malawi, the first public health measures such as lockdowns or the use of masks were arrived at using heuristics based on experiences and practices in other countries. The COVID-19 situation, and its unique, unknown characteristics, meant that leaders relied mostly on heuristics to make decisions and at times doctors too used heuristics for critical treatments.
But sometimes, using heuristics produces negative effects. I described above the situation in Malawi where, when trying to mitigate one type of gender bias by banning large gatherings or closing markets or schools to protect men, the result was to exacerbate other gender biases, like women losing access to income and education or experiencing higher rates of pregnancy and domestic violence, and to further aggravate existing constraints that apply to more vulnerable populations such as women, children, the elderly.
Lessons Learned and Further Questions
Lack of representativeness in data is a concern
For an algorithm to be effective, its training data must be representative of the communities that it may impact. In classical AI, heuristics play an important role in providing algorithms with those ‘rules of thumb’ that allow a solution to be reached when the only other option would be trial and error. In the era of machine learning and big data, we expect to have sufficient information in the data to limit the use of heuristics. For an algorithm to be effective, its training data must be representative of the communities that it may impact.
Data stratification can reduce biases
During COVID-19, it was common initially to report cumulative numbers – or total numbers of people infected over a period of time without looking at differences among sub-groups such as by sex or age or location. Treating all cases as being the same in this way. exacerbated the bias it produced in people’s minds and behaviour. Later on, data proved more insightful when looked along specific dimensions. This revealed that specific groups of people such as diabetics were at higher risks of infection or complications. Hence data stratification is an important technique to be used in both data collection and data analysis to reduce biases in data and decision-making.
More data does not always reduce bias
Could a large / exponential increase in health data reduce biases and improve representativeness? In theory this should be the case, but in reality, this isn’t always true. For example, in Malawi and Kenya, telephone surveys, which were frequently used during Covid-19, tend to capture more the views of men who have a higher phone ownership than women. Collecting more data does not always lead to a reduction in bias in the data.
Data scientists should not ignore differences
Covid-19 revealed a greater need to look for biases and assumptions that underlie human action globally, not by smoothing biases and differences but by revealing them. An AI chess tutor would be insensitive and less intelligent if it were to train women in the same way as men are trained. A data scientist analysing Covid-19 data should not ignore differences that are there whether one likes them or not.
Big data is dynamic, it has multiple dimensions, it is full of covariates (variables that researchers can’t control). So far, there has been no breakthrough in efforts to effectively reduce bias. We have effective methods for reducing noise but not biases. We need to continue this conversation.
The power of AI is in continuously fine-tuning its preconditions by learning from data. Heuristics can guide that finetuning. In Malawi, my work is to develop AI tools that integrate ‘heuristics’ by piecing together knowledge and decision processes that incorporate cultural differences in the best way I can.
Frankly, I can sympathise with AI systems. They need heuristics, but at the same time, they must reduce biases. They must have their cake and eat it.
*GAL stands for Gender Action Learning.
This post was written by Amelia Taylor, PhD, Senior Lecturer in AI at the Malawi University of Business and Applied Sciences, @LinkedIn, @GitHub, and is licensed under a CC BY 4.0 license. © 2023 Amelia Taylor.